1.Introduction to AIoT and Autonomous Vehicles
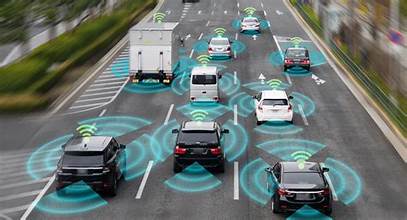
When devices we use in everyday life are getting increasingly smarter, the potential ways to utilize them are also increasing.
Due to increased interaction with people and other physical objects, IoT provides a great opportunity for collecting vast amounts of data. Artificial Intelligence (AI) applications can enable real-time analysis of the data that IoT devices have collected, helping devices to take immediate action based on the input data.
The combined power of AI and IoT, promises to unlock unrealized customer value in a broad swath of industry verticals such as edge analytics, autonomous vehicles, personalized fitness, remote healthcare, precision agriculture, smart retail, predictive maintenance, and industrial automation.
The autonomous vehicle segment is the fastest growing segment in the automotive industry. Artificial Intelligence is indeed the most important and sophisticated component of self driving vehicles (Carmody, Thomas, 2019).
The automated/autonomous vehicles, IoT, and artificial intelligence(AI) connected systems are increasingly relying on information that is 6.2 Automated Vehicles Connectivity Domains 257 exchanged to perform and conduct their safety-critical operations. Keeping such systems (and the data within) trustworthy, secure, safe, and private for the required cases is a critical element for the acceptance and adoption of such autonomous systems.
Intelligent connectivity enables new transformational capabilities in the mobility and transport sectors. The networks used for connecting IoT devices and vehicles must be ultra-reliable, as many critical tasks are executed remotely, and must rely on cost-effective edge infrastructure to enable low latency and scaling.Connectivity is, therefore, necessary for such services to work optimally. Intelligence enables the enhancement of user experiences through multi-access edge computing using, augmented reality (AR) and virtual reality (VR) technologies.
IoT devices and technologies can support automated/autonomous driving functions differently and enhance these functions for different use cases.
2.Integration of AIoT in Autonomous Vehicles
The abundance of sensors generating real-time data poses a significant challenge, necessitating intelligent processing capabilities. In contemporary automobiles, AI plays a crucial role not only in the central processing unit but also in numerous electronic control units (ECUs).
Given its extensive application in various fields, including robotics, integrating AI into automotive systems is a natural progression, promising to revolutionize autonomy. The underlying potential lies in AI and perception technologies enhancing safety and predictability, thereby yielding advantages such as improved fuel efficiency, enhanced comfort, and greater convenience (Sagar and Nanjundeswaraswamy, 2019).
A vehicle with automated features must have established reliable interactions with different domains that are interlinked through devices and systems. The
whole ecosystem relies on the interaction among the onboard units (OBUs),roadside units (RSUs), and vulnerable road users (VRUs). Intelligent sensors and actuators in the vehicles, roads and traffic control units in the infrastructures collect various information to serve enhanced automated driving (AD).
- Autonomous vehicles have neural networks and specific algorithms. These are Artificial Intelligence (AI) and Machine Language (ML) based object detection algorithms. These serve to collect data, analyze objects, and make accurate decisions while on the road. These features also enable these intelligent machines to provide solutions to problems occurring in advance of real time by predicting events through the swift processing of data.
- embedded algorithms help automobiles to determine and suggest alternative routes based on real-time traffic conditions.
- autonomous vehicles with enhanced AI features like personal AI assistants, radar detectors, and cameras, all of which serve to prioritize security.
- Self-driving cars learn about traits exhibited by the driver like driving speed, preferred car temperature, driving mood, observance of traffic signs, regular songs, or favorite radio stations. By rating driving skills, the autonomous vehicles will help to change bad driving behaviors and habits.
3.Automated Vehicles Connectivity Domains
A vehicle with automated features must have established reliable interactions with different domains that are interlinked through devices and systems.
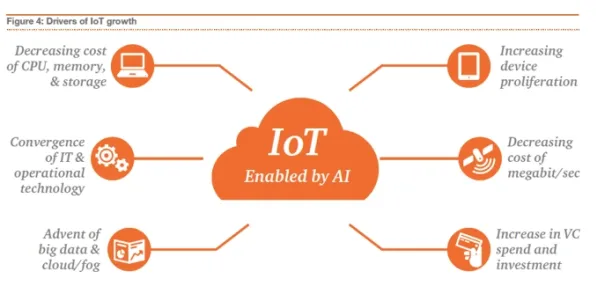
• Vehicle-to-infrastructure (V2I) communication is defined as the wireless exchange of information between vehicles and the roadside units of the infrastructure, such as traffic, road and weather condition alerts, traffic control, upcoming traffic lights information, or parking lot information.
• Vehicle-to-network (V2N) communication is the wireless exchange of information between vehicles and cellular networks, used for value-added services such as traffic jam information and real-time routing or available charging stations for electric vehicles (EVs).
• Vehicle-to-cloud/Edge (V2C) communication is defined as the wireless exchange of information between vehicles and the cloud or edge computing centres, for instance, used for tracking and usage-based insurance.
• Vehicle-to-Grid (V2G) communication is wired and/or wireless exchange of information between electric vehicles and the charging station/power grid for such as battery status and correct charging and
energy storage and power grid load/peak balancing.
• Vehicle-to-vehicle (V2V) communication is defined as the wireless exchange of information between vehicles about, for instance, the speed and position of surrounding vehicles.
• Vehicle-to-Pedestrian (V2P) communication is the wireless exchange of information between vehicles and vulnerable road users (VRUs) for safety-related services.
• Vehicle-to-home (V2H) communication is the wireless exchange of information between vehicles and a fixed or temporarily home, for instance, used for real-time routing. 6.2 Automated Vehicles Connectivity Domains 259
• Vehicle-to-Device (V2D) communication is wired and/or wireless exchange of information between the vehicle and IoT devices either inside or outside the vehicle.
• Vehicle-to-Maintenance (V2M) communication is the wireless exchange of information between the vehicle and the vehicle condition responsible (automotive manufacturer or repair shop), including vehicle condition monitoring, predictive maintenance notification or alerts.
• Vehicle-to-Users (V2U) communication is the wired/wireless exchange of information between the vehicle and its current user, including situational details.
• Vehicle-to-Owner (V2O) communication is the wireless exchange of information between vehicles and its owner. Use cases may be vehicle rental, fleet management, freight tracking, etc.
4. AI Applications in Vehicles
- Sensor Data Processing:
There are numerous sensors providing data for the central computer of the vehicle during operation. One of the main tasks is to detect and identify objects ahead and around the vehicle. Artificial neural networks (ANN) are the algorithms typically used for this task. Another term for this field is deep learning because a neural network contains many layers that contain many nodes.
- Path Planning:
The convergence of AIoT in path planning caters to the dynamic nature of navigation, where an array of sensors constantly provides critical environmental information. These sensors, integrated into the vehicle’s architecture, continually collect data regarding road conditions, traffic patterns, and surrounding obstacles.following definition of path planning is given: ”path planning for AVs enables self-driving vehicles to find the safest, most convenient, and most economically beneficial routes from point A to point B by using the previous driving experiences which help the AI agent make much more accurate decisions in the future”
- Path Execution:
Path execution involves several key steps facilitated by Path Planning AIoT:
- Real-time Sensor Data Collection: Autonomous vehicles are equipped with an array of sensors such as lidar, radar, cameras, and GPS units, continuously gathering data about the vehicle’s surroundings. Path Planning AIoT systems collect, process, and analyze this data in real-time to construct an accurate representation of the vehicle’s environment.
- Path Planning Algorithms: AI-powered path planning algorithms use the data collected from sensors to determine the optimal route for the vehicle to reach its destination. These algorithms take into account various factors such as traffic conditions, road obstacles, speed limits, and safety considerations.
- Dynamic Path Adjustment: Path Planning AIoT systems are capable of dynamically adjusting the vehicle’s path in response to changing environmental conditions or unexpected obstacles. This adaptive capability ensures that the vehicle can navigate safely and efficiently in complex and dynamic environments.
- Communication with Control Units: Path Planning AIoT systems communicate with the vehicle’s control units, including the central processing unit and electronic control units (ECUs), to execute the planned path. This communication facilitates real-time adjustments to vehicle speed, steering, and braking to follow the planned route accurately.
- Integration with Navigation Systems: Path Planning AIoT systems are seamlessly integrated with onboard navigation systems, providing drivers and passengers with real-time updates on the vehicle’s progress and estimated time of arrival. This integration enhances the overall user experience and ensures smooth coordination between the autonomous vehicle and its occupants.
- Monitoring Vehicle’s Condition:
Predictive maintenance employs monitoring and prediction modelling to determine the condition of the machine and to predict what is likely to fail and when it is going to happen.It tries to predict future problems, not problems that already exist.he algorithms can use on-board and off-board data to make decision for predictive maintenance.The machine learning algorithms used for this task are classification algorithms like logistic regression, support vector machines and random forest algorithm.
5. Benefits and Challenges of AIoT in Autonomous Driving
5.1.Benefits of Autonomous vehicles
Autonomous Vehicle Benefits Leveraging AIoT Advancements in Technical Terms:
- Enhanced Sensor Fusion: Integration of AIoT technologies enables sophisticated sensor fusion algorithms, combining data from diverse sensors such as lidar, radar, cameras, and GPS units. This fusion enhances environmental perception accuracy, allowing for robust decision-making in dynamic driving scenarios.
- Optimized Path Planning: AIoT-powered path planning algorithms leverage real-time data analytics to dynamically compute optimal routes considering factors like traffic congestion, road conditions, and weather patterns. This optimization minimizes travel time, enhances fuel efficiency, and ensures smoother navigation.
- Dynamic Obstacle Detection and Avoidance: AIoT systems facilitate advanced obstacle detection and avoidance mechanisms by continuously analyzing sensor data streams. Machine learning algorithms discern patterns and predict potential obstacles, enabling proactive navigation adjustments to ensure collision-free travel.
- Predictive Maintenance: AIoT integration enables predictive maintenance strategies by monitoring vehicle health metrics in real time. Anomalies in engine performance, tiretyre pressure, or battery health are detected early, allowing for preemptive maintenance actions, minimizing downtime, and optimizing fleet operations.
- Efficient Energy Management: AIoT-based energy management systems optimize power consumption by intelligently controlling vehicle subsystems. Smart algorithms adapt HVAC (Heating, Ventilation, and Air Conditioning) settings, powertrain configurations, and auxiliary systems to maximize energy efficiency without compromising passenger comfort or safety.
- Advanced Driver Assistance Systems (ADAS): AIoT enhances ADAS capabilities by integrating adaptive cruise control, lane-keeping assist, and automated emergency braking functionalities. These systems leverage AI-driven algorithms to enhance driver safety, reduce the likelihood of accidents, and improve overall driving experience.
- Data-driven Decision Making: AIoT platforms facilitate data-driven decision-making by aggregating and analyzing vast volumes of vehicle telemetry data. Predictive analytics algorithms identify trends, anticipate maintenance needs, and optimize vehicle performance, leading to enhanced reliability and cost savings.
- Compliance and Regulatory Alignment: AIoT systems aid in ensuring compliance with regulatory standards and safety requirements by continuously monitoring vehicle operations and performance metrics. Real-time reporting and auditing capabilities facilitate regulatory alignment and support autonomous vehicle certification processes.
5.2.Drawbacks of autonomous vehicles
The challenges in developing AI systems for something as complex as a self driving vehicle are many. The AI has to interact with multitude of sensors and has to use data in real time. Many AI algorithms are computationally intensive and are therefore hard to use with CPUs that have memory and speed restrictions. Modern vehicles are an example of real time systems that have to produce deterministic results in the time domain. This is related to achieving safety while driving the vehicle. Complicated distributed systems like these require a lot of internal communications that are prone to latency which can disturb the decision making of the AI algorithms. In addition there is the issue of power consumption of the software running in the car. The more intensive AI algorithms consume more power, which is an issue, especially for electric vehicles that depend only on the charge of the battery.
Though one of the most anticipated technologies in this century, AI and autonomous vehicles have been associated with a number of problems:
- Autonomous vehicles are limited to more narrow situations and clearer weather. Just like the human eyes, sensors do not do well in fog, rain, or snow.
- Autonomous vehicles rely on maps and sensors to function effectively. Unfortunately, these maps have limited test areas at the moment. Creating and maintaining maps for self-driving cars is a difficult and time-intensive process and one that is yet to happen. Its test areas will also need to be increased.
- Autonomous vehicles controlled by AI robots cannot engage in complex social interactions with other drivers, cyclists, and pedestrians. These situations require generalized intelligence and common sense to navigate, qualities that robots do not possess at the moment.
- The working mechanism of smarter and more connected autonomous vehicles can suffer from cyber attacks which would disrupt their systems and operational processes. When this happens, commuter stress, delayed traffic flow, collisions, accidents, and even loss of human lives become inevitable.
Enhancing Safety and Efficiency with AIoT
- Collision Avoidance Systems: AIoT-enabled collision avoidance systems utilize data from onboard sensors to detect and respond to potential collision risks. Machine learning algorithms analyze sensor data patterns to predict and prevent accidents, enhancing overall road safety.
- Adaptive Cruise Control (ACC): ACC systems, powered by AIoT, adjust vehicle speed based on traffic conditions and surrounding vehicles’ movements. By maintaining a safe following distance and automatically adapting speed, ACC enhances safety and reduces the likelihood of rear-end collisions.
- Traffic Management and Optimization: AIoT solutions integrate with traffic management infrastructure to optimize traffic flow and reduce congestion. By analyzing real-time traffic data and coordinating with traffic signals, autonomous vehicles can navigate efficiently, minimizing travel times and emissions.
- Driver Assistance Systems: AIoT-enabled driver assistance systems provide real-time alerts and interventions to prevent driver errors and mitigate risks. Features such as lane departure warning, blind-spot detection, and automatic emergency braking enhance driver safety and reduce accident rates.
- Route Optimization: AIoT-based route optimization algorithms consider various factors such as traffic congestion, road conditions, and weather forecasts to determine the most efficient travel routes. By avoiding traffic hotspots and road hazards, vehicles can reach their destinations faster and with lower fuel consumption.
- Enhanced Vehicle-to-Infrastructure (V2I) Communication: AIoT enables seamless communication between vehicles and infrastructure elements such as traffic lights, road signs, and pedestrian crossings. This connectivity facilitates safer interactions between vehicles and their surroundings, reducing the risk of accidents.
- Behavioral Analysis and Risk Assessment: AIoT solutions analyze driver behavior and vehicle telemetry data to assess risk levels and identify potential safety hazards. By providing actionable insights, these systems help fleet operators implement targeted interventions to improve driver safety.
- Emergency Response Coordination: In the event of an accident or emergency, AIoT systems can automatically alert emergency services and provide critical information such as vehicle location and severity of injuries. This rapid response capability can significantly reduce emergency response times and improve outcomes for accident victims.
Path to the Future
In the crypto world, blockchain technology makes use of mathematical logic and algorithm to create an autonomous system that is both transparent and immutable. Here in the automotive world, it is expected that the combination of blockchain technology and self-driving cars will create a far better autonomous system that would increase the transparency and accuracy of decisions made by these cars.
Car tech giants are working hard to implement natural conversational AI within vehicles, which will utilize speech recognition, natural language understanding, speech synthesis, and smart avatars to boost comprehension of context, emotion, complex sentences, and user preferences. In the future, AI will be engaged in improving vehicle safety, performance, and efficiency, addressing health hazards and environmental issues. It could also be used to create cars that can communicate with each other and with other road users.